Professor Gareth Williams
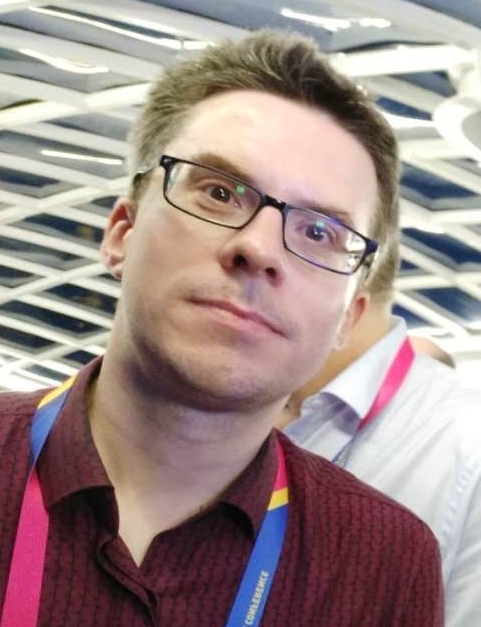
Biography
Gareth received a MChem (Hons) degree from the University of Oxford in 2002. He remained in Oxford for a DPhil (PhD), which was completed in 2005. He then worked as a post-doctoral researcher and in science program management. In September 2010 he joined London Metropolitan University as a Senior Lecturer in Pharmaceutical Science, and in November 2012 was appointed to the UCL School of Pharmacy as a Lecturer in Pharmaceutics. He was promoted to Associate Professor in 2016 and to full Professor in 2020, when he also became Head of Pharmaceutics. Gareth leads a group of around 20 researchers applying inorganic and polymer-based nanomaterials to overcome drug delivery challenges.